HF-FCN: Hierarchically Fused Fully
Convolutional Network for Robust Building
Extraction
Tongchun Zuo Juntao Feng Xuejin Chen*
University of Science and Technology of China
Asian Conference of Computer Vision 2016 |
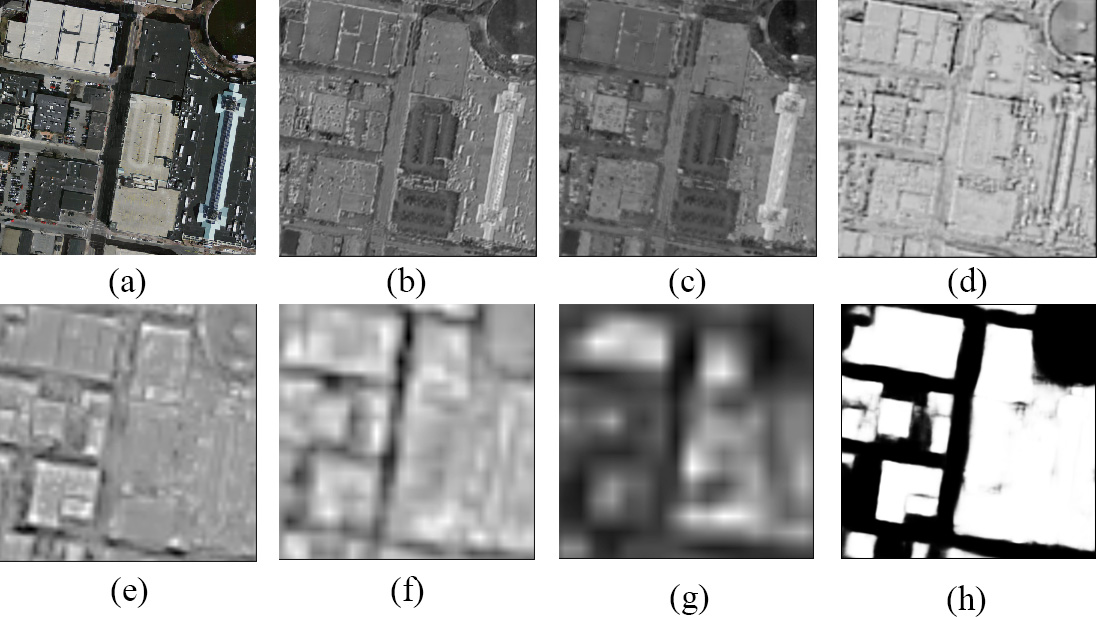
|
Figure 1: (a) Input aerial image. (b - c) Feature maps generated from shallow layers with fine spatial resolution but low
level semantic information. (d - e) The feature maps at middle layers
correspond to certain intermediate-level features. (f - g) Deep layers generate coarse feature
maps with high-level semantic information. (h) Integrating all these feature
maps, we get predicted labelling map. |
|
Abstract: |
Automatic building extraction from remote sensing images plays an important role in a diverse range of applications. However, it is
significantly challenging to extract arbitrary-size buildings with largely
variant appearances or occlusions. In this paper, we propose a robust
system employing a novel hierarchically fused fully convolutional network (HF-FCN), which effectively integrates the information generated
from a group of neurons with multi-scale receptive fields. Our architecture takes an aerial image as the input without warping or cropping it
and directly generates the building map. The experiment results tested
on a public aerial imagery dataset demonstrate that our method surpasses state-of-the-art methods in the building detection accuracy and
significantly reduces the time cost. |
|
Results: |
|
Figure 2: An example of the triplet images. |
|
|
Figure 3: The relaxed precision-recall curves from different methods with two slack parameters |
|
|
Figure 4: (a) Input images. (b) Results of Mnih-CNN+CRF [1]. (c) Results of Saitomulti-MA&CIS [2]. (d) Our results. Correct results (TP) are shown in green, false
positives (FP) are shown in blue, and false negatives (FN) are shown in red. |
|
Acknowledgements: |
We would like to thank the anonymous reviewers. This
work was supported by the National Natural Science Foundation of China (NSFC) under Nos. 61472377 and 61331017, and the Fundamental Research Funds
for the Central Universities under No.WK2100060011. |
|
References: |
[1] Mnih, V.: Machine learning for aerial image labeling. Doctoral (2013)
[2] Saito, S., Yamashita, Y., Aoki, Y.: Multiple object extraction from aerial imagery
with convolutional neural networks. Journal of Imaging Science & Technology. (2016) |
|
BibTex: |
@article{Zuo-ACCV16-HF-FCN,
author = {Tongchun Zuo, Juntao Feng, Xuejin Chen},
title = {HF-FCN: Hierarchically Fused Fully
Convolutional Network for Robust Building
Extraction},
conference = {Asian Conference of Computer Vision},
year = {2016}
} |
|
Downloads: |
Disclaimer: The paper listed on this page is copyright-protected. By clicking on the paper link below, you confirm that you or your institution have the right to access the corresponding pdf file. |
|